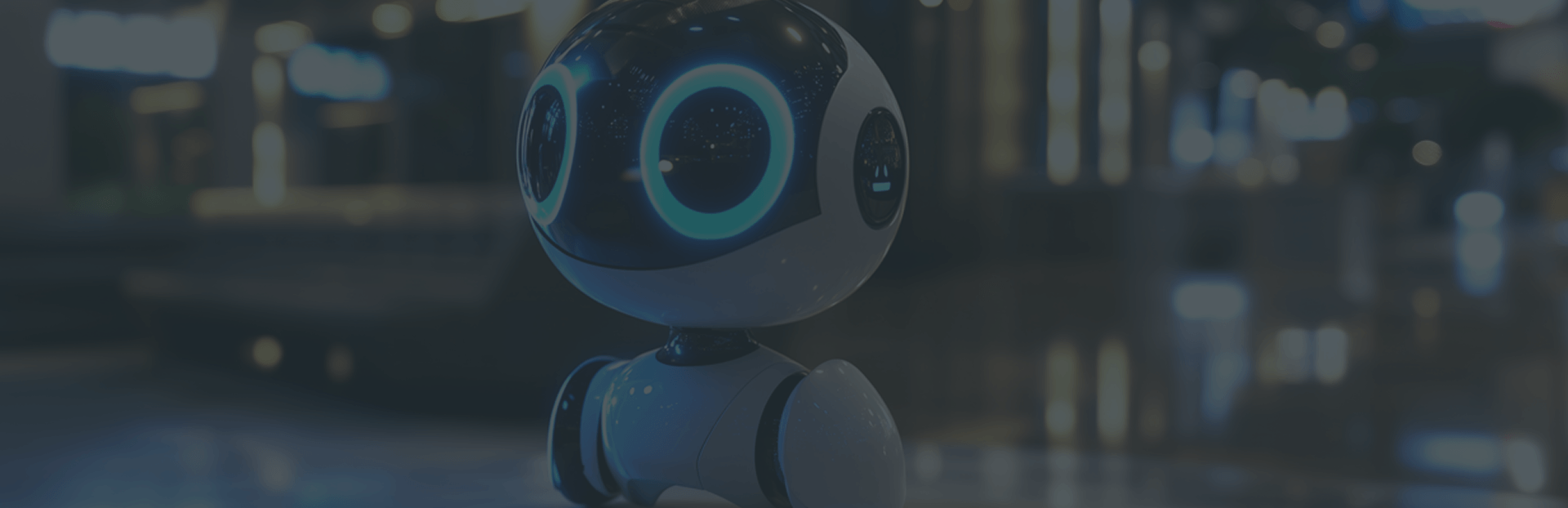
Author’s Thoughts
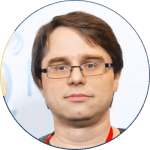
"Silk Data team shares common opinions on great perspectives of developing and using intelligent AI assistants. The blog post explores the topic, analyzing the main principles of present AI agents’ progress and describes certain considerations connected to their usage."
Nikolai Karelin
AI is regularly ranked as one of the most fast-growing spheres with a wide field of possibilities in its development.
Currently, it provides a huge variety of subfields, each of them devoted to specific aspect or application case. One such application is AI agents – intelligent assistants meant to help humans to perform certain tasks.
This article investigates the reason AI agents are becoming so popular and explains which benefits (and problems) companies can get from using them.
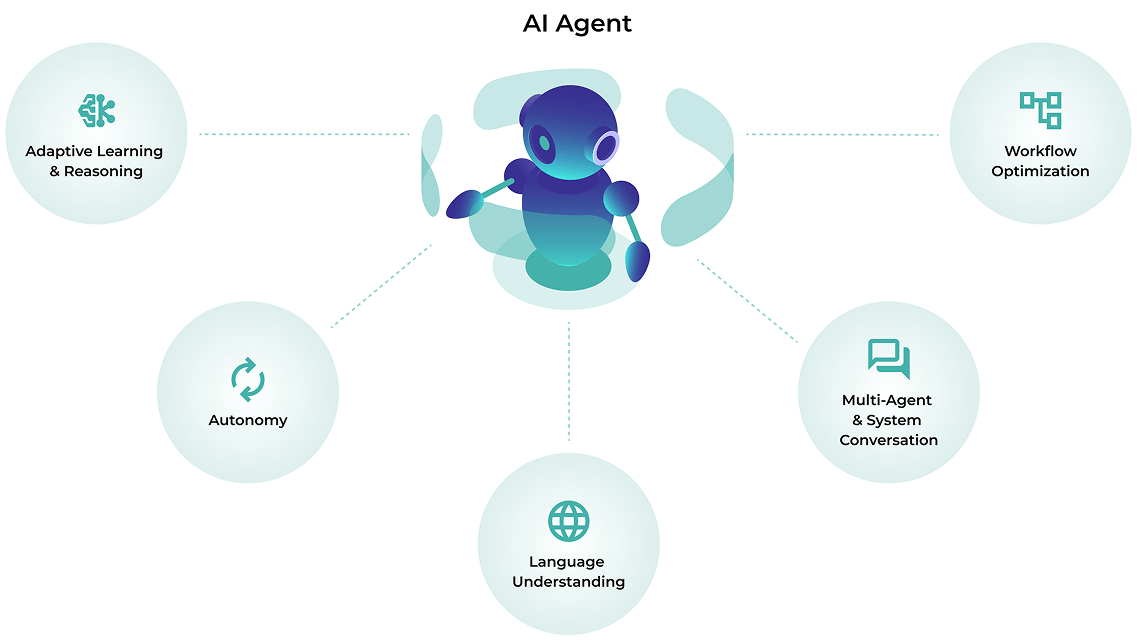
What Is an AI Agent?
Artificial intelligence agent is defined as a program or software developed with the aim to assist specialists in performing certain tasks.
The ways of assistance are defined by developers of agent AI and can include content generation or specific business processes automation, such as intelligent contract processing and text summarization.
Why is it necessary? To properly classify the document on its type, purpose or insights.
However, their function isn’t limited to performing routine tasks. The AI agents have already proved themselves to be capable of performing complicated analytical tasks and enhancing advanced decision making.
According to Deloitte’s report, by the end of 2024 about 26% of world companies have already tested agentic AI capabilities of performing operations autonomously, which means that is without permanent human intervention and control.
AI agents examples can be represented by chatbots that perceive user queries or input data, analyzes them and provide optimal decisions or advanced predictions. AI agent can even provide an action plan, step-by-step, to achieve the goal the user describes in an input.
This planning and reasoning feature along with the possibility of self-reflection answer the question: ‘What is agent in AI?’ and define the agentic approach in AI development. In other words, AI tools which use this approach are close to a human working process imitation – they can set the goals and achieve solutions with the following self-correction.
Now it is commonly accepted that 2025 will be the year of AI agents as more businesses are ready to invest additional resources in the industry.
Key Principles of AI Agents
Field Evolution
The common tendency of the past few years is in spreading of agentic AI based on the usage of large language models (LLMs) technology.
Intelligent AI assistants leverage the capabilities of LLMs to perceive, understand and generate human-like content. AI agents’ reinforcement by LLM leads to certain key points in the sphere’s development.
We can highlight the most crucial ones:
Natural Extension of Traditional Chat-bots and RAG Applications
Applying large language models means that AI assistants have made a step forward compared to traditional non-agentic chatbots and applications based on retrieval-augmented generation (RAG).
As these chatbots and applications used to rely on rule-based systems and models based on intentional analysis, their capabilities of deep contextual and semantic understanding of the human language were rather limited.
Usage of LLM certainly improves the capabilities of chatbots. They are able to continuously learn and adapt to multiple use cases, they're able to primarily understand the context and provide more natural responses, thanks to being trained on much larger amounts of data. However, despite the possibility of processing and generating human-like content, non-agentic LLM-based chatbots are still limited in solving certain tasks, as they lack memory and reasoning abilities. This fact leads to the second key point.
LLM as a Universal Brain
All LLM-based systems can provide answers the user is likely to receive, but they demonstrate rather poor performance on unique queries, and the lack of memory holds the ability to learn from mistakes.
On the contrary, agentic AI chatbots are capable of advanced learning. They can align to user expectations over time and quickly change the initial responses. Moreover, new versions of popular LLMs have reasoning ability: split the complex task into simpler steps, either mental, like decisions or computations or activities, like using external tools.
We can say that the principle of AI agents’ work is, at some point, similar to the human brain, as it’s able to adapt to new inputs and correct its own mistakes.
External and Internal Data
The core of all non-agentic LLM systems lies in a long process of model training, when it is fed with the huge amount of data (sometimes, billions or even trillions of text tokens) that allows the model to understand the language and the concept of the tasks it has to perform.
It seems effective, yet rather time-consuming, as in many cases the following approach is enough for performing a standard task.
At the same time, principles of agentic AI tools lie in wide usage of both external and internal data.
This hybrid approach allows the model to efficiently permanently learn and improve its own performance. For instance, an AI agent can create forward-thinking strategies (performing as mentioned above universal brain) and correct them over time through perceiving and analyzing side sources (websites, open-source surveys, etc.) and internal materials (for example, corporate database changes).
It is worth noticing that the nature of an agentive AI allows to perform all the described operations in autonomous way with minimum user intervention.
Agentic LLMs and chatbots based on them seem to be the new stage of industry’s evolution. The following table provides a comprehensive overview.
Criteria | Traditional Non-LLM-Based Chatbots | Non-Agentic LLM-Based Chatbots | Agentic LLM-Based Chatbots |
---|---|---|---|
Human Language Perception, Processing and Generation | Rule-based processing with limited natural language understanding and rigid response patterns | Advanced natural language generation with fluid, human-like responses which lack autonomy | Highly adaptive, real-time language processing with goal-oriented conversation flow |
Rule-based processing with limited natural language understanding and rigid response patterns | Shallow context awareness, struggles with maintaining long conversations | Strong contextual understanding with memory over short interactions | Deep, persistent memory and nuanced comprehension across long-term interactions |
Memory | Limited or no memory; relies on predefined flows | Short-term memory, sometimes retains context within a session | Long-term memory, capable of recalling past interactions for improved personalization |
Reasoning | Basic ‘if-then’ logic with no real inference capabilities | Advanced reasoning using statistical patterns but lacking structured, goal-driven logic | Strong reasoning abilities with structured multi-step thinking and decision-making |
Predictive Planning | No predictive planning, as responses based on predefined rules | Limited foresight, as can infer possible next steps but doesn't plan proactively | Actively formulates plans, sets objectives and adapts strategies dynamically |
Self-Correction | No self-correction; errors require manual intervention | Can rephrase and refine responses within a session but lacks persistent learning | Self-evaluates, learns from mistakes and refines strategies over time |
Such advanced capabilities of AI agents are possible in no small part thanks to the usage of different frameworks, workflow builders and platforms (LangChain, LangGraph, Vertex AI, etc.) that help to build and maintain reasoning logic, monitor model performance and improve its accuracy.
How Can AI Agent be Integrated into Your Business?
Generative AI agents proved to be a promising field of AI, as many companies appear to be optimistic about using ChatGPT and its analogues in business. Through that, it is necessary to make an overview of how a combination of artificial intelligence and intelligent agents can be used in practice.
Marketing
Apart from cost reduction connected with freeing specialists from repetitive and time-consuming tasks, advanced AI assistants can be used in far more complicated tasks.
For instance, the reasoning feature of agentic AI tools allows them to build marketing strategies or content plans based on business capability data or make predictions based on data from thorough market research and analysis processed by the system.
Silk Data has a successful case of a related solution, when the marketing agency received intelligent LLM-based chatbot that could streamline team workflows.
Retail and Sales
The same practice can be applied for the spheres of sales, commerce and procurement. AI agents significantly improve forecasting (predicting future product demand based on historical and present data), help to create pricing and discount strategies (based on market situation and competitors' analysis) and create a set of recommendations that can be proposed to the customers.
It already demonstrates its usefulness. According to Salesforce survey, in 2024 sales teams utilizing AI agents demonstrated a 25% higher revenue growth compared to those who still don’t use it (83% and 66% accordingly).
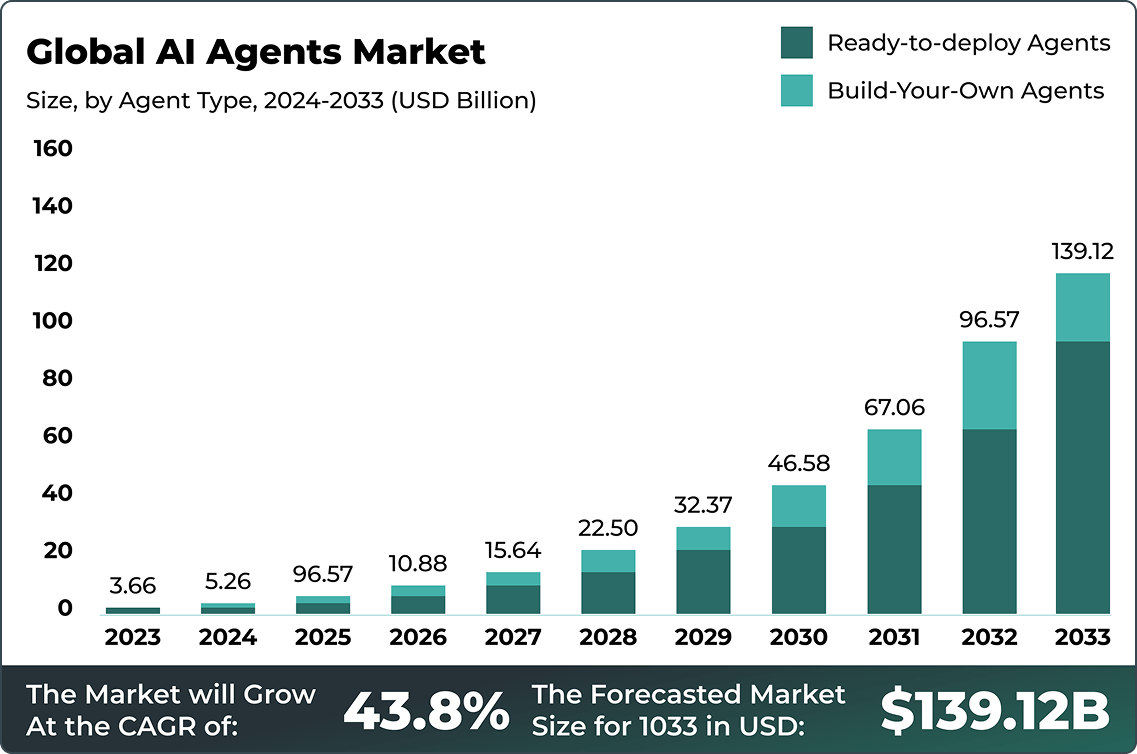
Regarding the procurement sphere, AI agents can be useful while dealing with customer applications. Silk Data has already developed an AI solution that helped a procurement company to analyze email messages, classify them and improve decision making processes.
Education
There are lots of AI tools already used in education, but they are commonly focused on AI and plagiarism detection or on performing simple routine tasks.
Agentic AI being focused on solving personalized tasks can provide efficient and unique learning experience. Practically, it can be implemented in the form of an AI tutor that can analyze students' progress and adjust the study program according to their successes and failures.
Ecology
As environmental issues require permanent monitoring, usage of AI agents seems to be proper solution to automate the process.
In perspective, such software can be used in online monitoring of weather conditions or tracking of energy consumption and waste management.
Important Considerations on AI Agent Development and Implementation
The benefits the AI agents can provide are tempting for many companies. However, like any explicit innovation, the combined usage of artificial intelligence and intelligent agents provides a set of concerns that should be kept in mind.
For example, Craig Le Clair, an analyst at Forrester Research declared:
We see several points regarding this concern.
Common Uses-Cases vs. Custom Solution
Though intelligent AI assistant seems to be extremely useful, there are many cases when the usage of agentic AI appears to be excessive.
If you need to acquire the LLM-based tool to deal with common tasks such as automation of repetitive operations or non-specified content generation, it’s better to focus on simple solutions with relatively simple architecture.
At the same time, for specific cases when the AI tool must solve unique tasks or provide sophisticated solutions such as action plan building, agentive AI is a better option.
Hallucinations and Human-in-the-Loop
Even the most advanced LLM-based AI assistants can't guarantee 100% accuracy.
In some spheres, such as legal and finance, even miner mistakes can lead to sufficient money, time and reputation losses.
We say that the problem of model hallucinating can be primarily solved through special guardrails or regular fine-tuning while human specialists should review the results it provides.
Security and Data Access
As AI agents are meant to work both with external and internal data, data access and security concerns become vital. It can be connected both with common data security regulations alignment and the security of the model itself which can be harmed by insecure or poisoning data.
The possible solutions of the present days relate to adversarial testing to identify system’s weaknesses or regular auditing aimed to track and correct biases.
Moreover, some actions, such as access to bank accounts, should go through human acceptance on every transaction: for example, AI agent can find and prepare all information for a business trip, but the flight tickets, hotels etc. must be booked only after human review.
Harmful Outputs
The reasoning and memory ability of AI model guarantees a certain level of freedom and creativity in its work.
In some cases, however, it can lead to the generation of offensive or ethically inappropriate content along with content containing direct misinformation. The research analysis says that this point remains one of the most vital concerns for organizations to implement AI agents.
Most of these research papers can’t offer anything better than regular content moderation, and we can only agree with them.
2025 can truly become a year of AI agents. The advanced architecture and the usage of the most up-to-date frameworks and workflows and the following capabilities of such models make intelligent AI assistants rather perspective for application in different spheres.
However, this direction of AI is still developing, and not all the tasks can be delegated to AI without concern. There is much work to be done regarding agentic AI issue resolution which, we believe, is only a matter of time.
Our team will continue to observe and evaluate this direction of modern AI. Subscribe us on LinkedIn for more information or get in contact with our team if you have more specific questions or inquiries.
Our Solutions
We work in various directions, providing a vast range of IT and AI services. Moreover, working on any task, we’re able to provide you with products of different complexity and elaboration, including proof of concept, minimum viable product, or full product development.